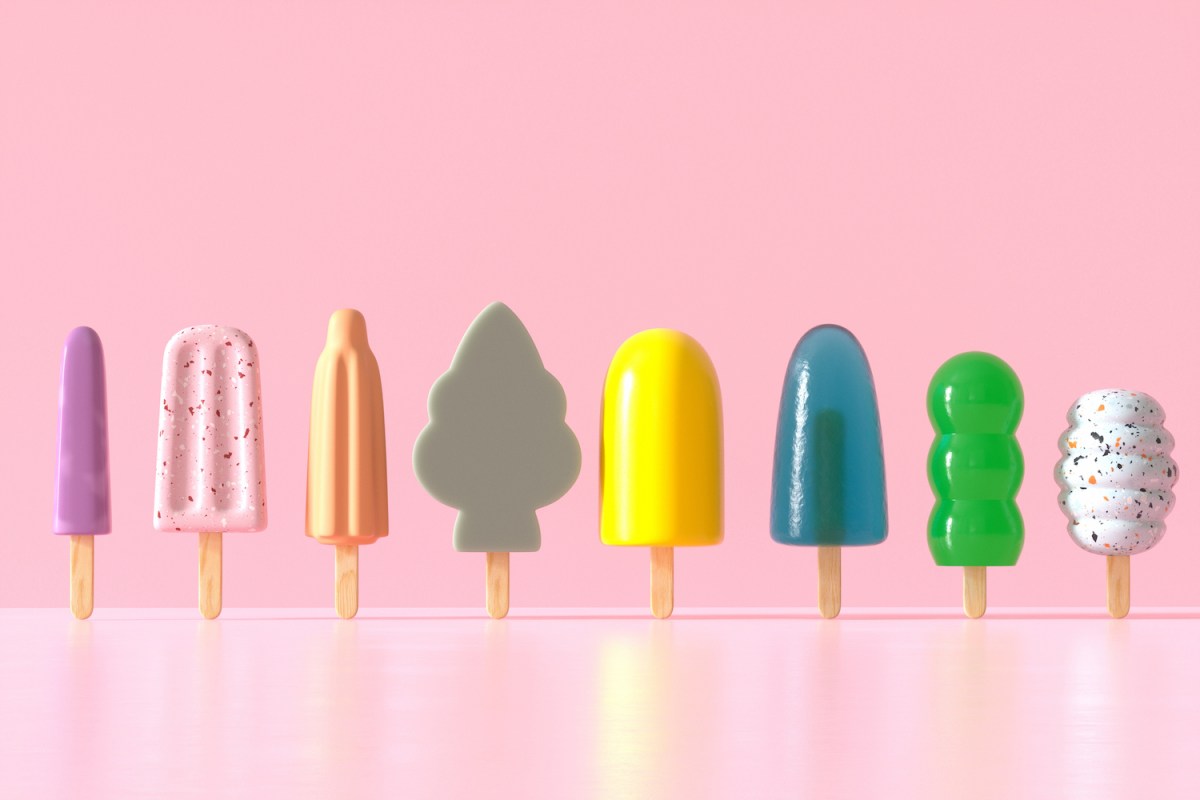
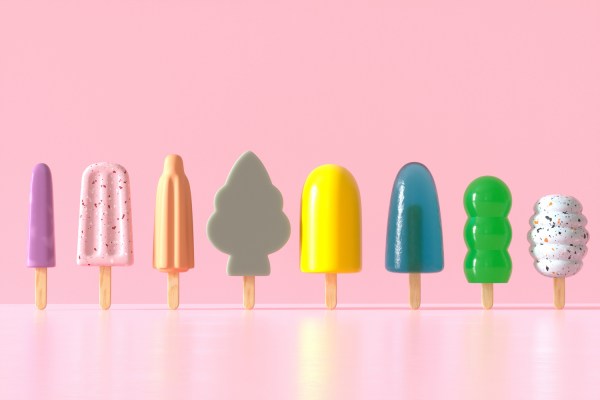
The launch of ChatGPT in November of 2022 propelled our world into the Age of AI, and the tech industry will never be the same.
Nearly every pitch deck I’ve seen since December has had AI on the front two pages.
As with any emerging technology, however, venture capitalists like myself have had to quickly develop a strategy to separate the high-potential startups from those that are mostly hype or are likely to face insurmountable challenges that will prevent them from achieving venture scale.
Understanding that distinction requires fluency in the various layers of the generative AI value stack, determining which are ripe for investment and creating a due diligence strategy to evaluate the risks and opportunities of a given startup.
Specifically, generative AI is composed of:
- Data.
- Middleware.
- Fine-tuned specialized models.
- The cloud and infrastructure layer.
- Foundational models.
- The application layer.
Within this tech stack, there are a few areas that we think are especially investable and others that are more challenging for a seed-stage company to compete in. Here’s how we break it all down.
Areas we’re interested in
Data
One of generative AI’s greatest challenges — and thus one of its greatest areas of opportunity — is the accuracy and reliability of the information it provides. Today, generative AI models are built on massive datasets, some as wide and as broad as the internet itself, containing both relevant and useful information, and a whole lot of everything else.
We believe that the galaxy of generative AI applications that will emerge in the coming years will be composed of more precise data, or bits and pieces of different, more specialized models. Rather than casting a wide net, these specialized models will utilize proprietary data specific to a domain, which will help to personalize the output of the application as well as ensure accuracy.
There are a few areas that we think are especially investable and others that are more challenging for a seed-stage company to compete in.
Having proprietary data to infuse with foundational models — combined with the right middleware architecture — will result in these specialized models, which we believe will power the application layer that consumers and businesses interact with.
Middleware
Accompanying the data layer of the generative AI stack is middleware, which we define as tooling and infrastructure that supports the development of new generative AI applications and is the second part of our investment thesis in the sector.
Specifically, we are bullish on infrastructure and tooling companies that evaluate and ensure safety, accuracy, and privacy across model outputs; orchestrate inference across multiple models; and optimize incorporating proprietary data into large language models (LLMs).